Why You Need Real Time Right Now
Time is the enemy of forecasting
In our previous post, we discussed why demand forecasting matters and how an accurate demand forecast can mathematically and quantitatively improve your service level and/or inventory levels (cost). Now it is time to focus on the biggest uncertainty of them all. Time.
Advanced forecasting algorithms can help eliminate uncertainty through reducing variables that are “unknown” by understanding relationships at a scale that no human could comprehend. This helps turn the seemingly “unknown” in to a “known”. As we stated earlier, advances in math and cloud computing make finding these relationships much easier and can be computed almost instantaneously. However, the element of time is a tricky compounding factor. Even little unknowns become big over time as time compounds all errors and introduces more uncertainties.
The most certainty you have is in the precise moment you make an estimate. When you look ahead 1 day, 1 week or even 1-month (as most corporate S&OP) cycles still do – then you must start to rely less and less on facts and more and more on assumptions. Assumptions mean uncertainty and uncertainty means cost.
There are loads of examples of this. When I lived in Florida,
every September during hurricane season,
we would get the dreaded cone of uncertainty any time a hurricane formed. As below shows,
the further out you get, the
expected position gets wider. By day 5 - the uncertainty stretched over 500 miles – hardly accurate enough to do any planning of significance.
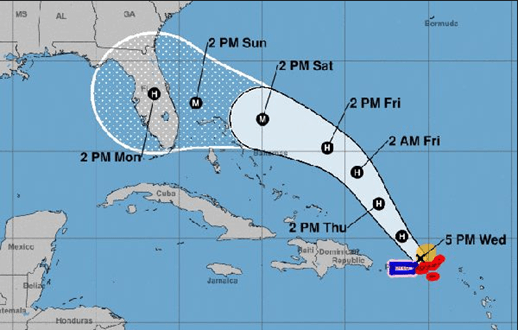
Would you wait 1 week for a forecast update on this?
Enter Real time forecasting (instant ML)
If you could look in real-time, everyday there are hundreds of variables influencing your demand. Conditions can change rapidly which requires models to be rebuilt based on live data (real sales, real stock availability, real weather conditions, promotions, pricing…. the list goes on). We have shown that, with machine learning, we can model many of these, but a static model is not great either (look what happened to all those companies that had rigid statistical forecasting models in the time of COVID). To obtain the best possible projection of demand, companies need to work on data as close to real-time as possible and recompute models based on what is happening now, not what we think might happen in a week or even what happened last week.
Let's look at a real example of a recent customer and a new product launch. This was a new product with little history, and the launch team made some best estimates of launch demand. As you can see, with instant ML we are able to remodel and re-predict the forecasts using the most real-time data from the launch. This provides a quick consensus of models and enables resources to be diverted to meet true demand. Sticking with the original model for longer would have created an almost certain inventory impact and huge bullwhip across the supply chain. Using real-time data ensures that we are keeping our variability down as low as possible, which enables us to keep our service level high and our cost down.
We closed off our last post with the following thought...
Know what you do not know costs you. (and there is a pretty accurate equation for this)
Well if you are reforecasting with an out of date model, or you are reforecasting without the latest available data - you are definitely leaving information that you know out of your model and forecasts. This is costing you money and/or impacting your service.
Next, we will start to explore how technologies can close these gaps – and make sure that you are providing your supply chain with the most accurate demand. That means leveraging all the variables you have (at the lowest possible level) when predicting your demand and doing it as fast and as often as you can.
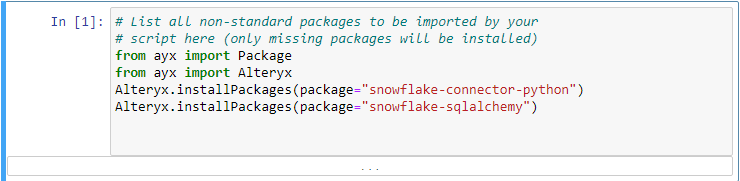
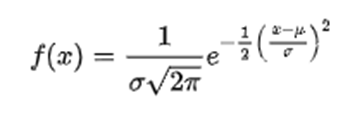
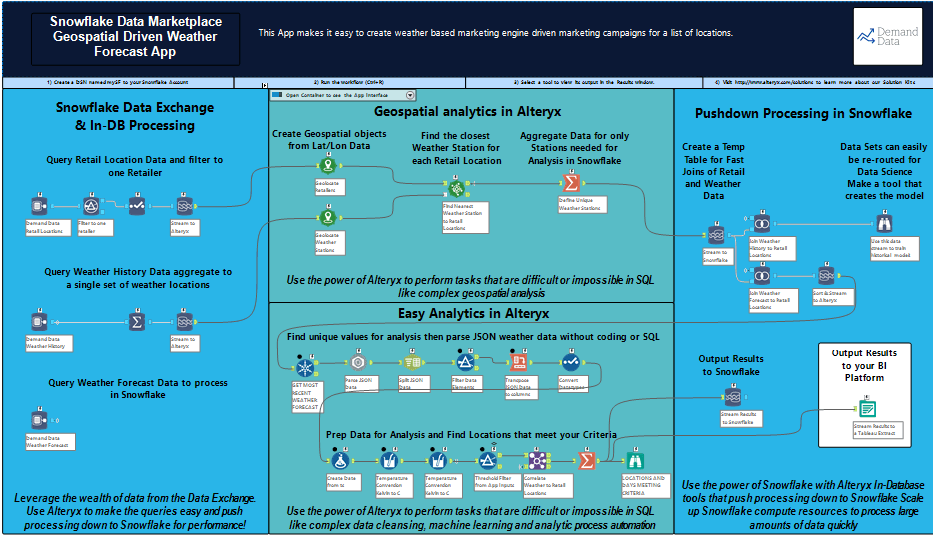